Inside the black box: AI must be explainable to be ethical
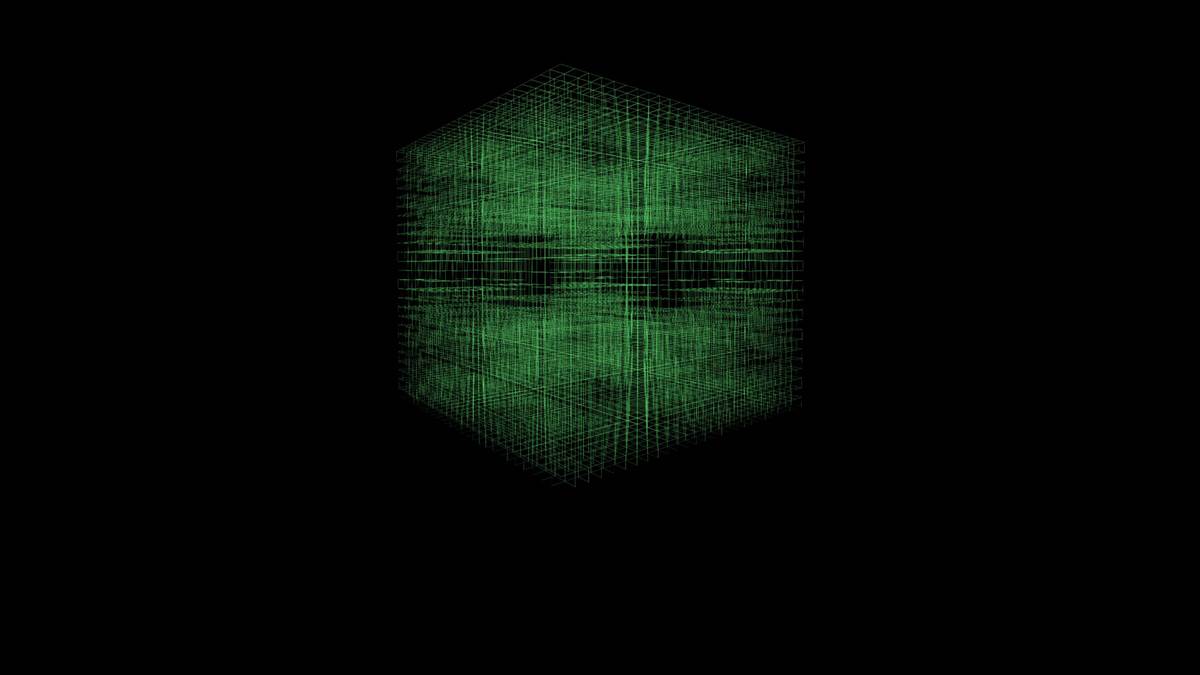
“Computers are programmed by people and just as liable to be coded with our faults, as our virtues.”
A fundamental transformation
Identify customers to personalize experiences
Monetize the customer journey
“Whether it is an oath, a product label or an ISO number, end-users will soon be able to pick an AI developer based on their adherence to ethical guidelines.”
Optimize and improve conversions at scale and over time
Get more insights from Thread
Read now