How can Google Cloud support B2B price optimisation and demand forecasting?
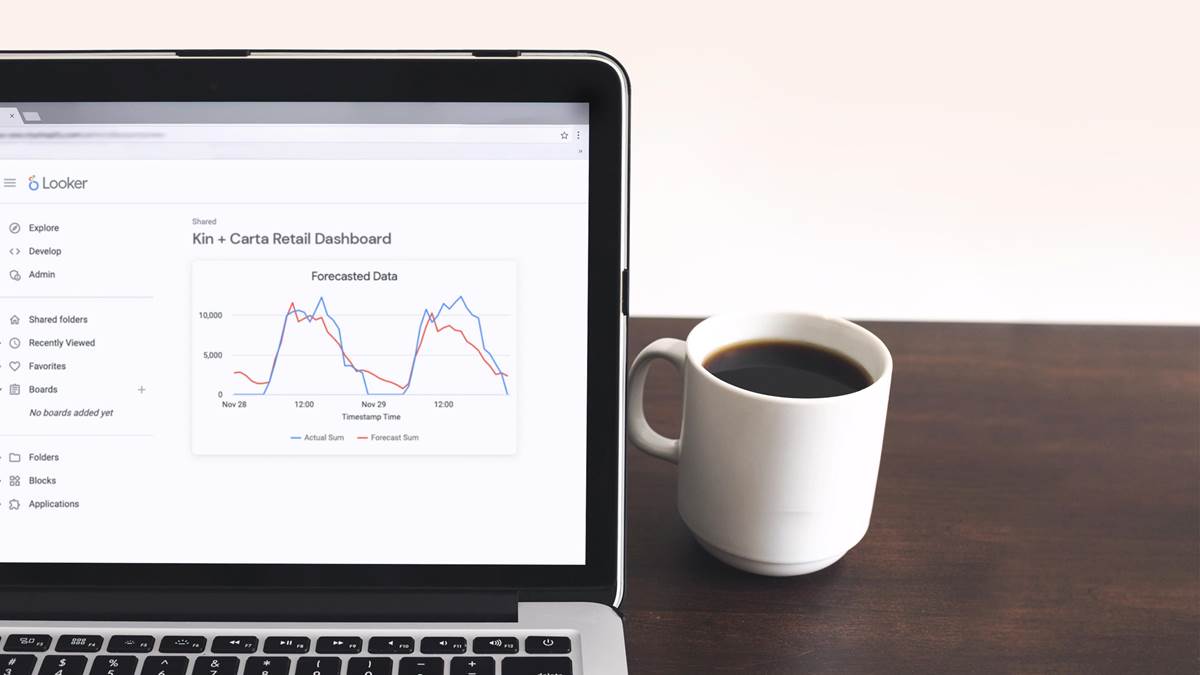
In Part 1 and Part 2 of this Supply Chain Optimisation series, set largely in a B2C context, we looked at the story behind how the price of my pair of jeans was being influenced through Machine Learning (ML) price optimisation, and how a retailer could extend this capability to also incorporate demand forecasting.
In Part 3 of our Supply Chain Optimisation series, we looked at what makes B2B different from B2C, and the challenges and opportunities that come with B2B. In this fourth and final installment, we look at how we can apply the same principles of price optimisation and demand forecasting in a B2B business operating model, and in the context of the increasingly challenging economic outlook B2B retailers are likely to face in 2022 (and beyond).
How do we provide optimised pricing to a B2B buyer when, as we covered in Part 3, this often has several intrinsic steps and dependencies?
In Part 1, when we explored the opportunities for B2C, we looked at how historical transactional data and other signals are imperative inputs for calculating the price of traditional consumer retail items. In B2B, there is a far greater degree of analysis and pricing science that goes into pricing optimisation. This means developing and deploying a system that enables a B2B retailer to reset price targets in real time at a customer/product level, based on actual facts, as well as developing a dynamic-pricing capability that places as much on people and processes as it does on analytics and technology. It is this additional level of complexity, and the need for simulations, which creates the opportunity for B2B retailers to make use of predictive analytics, and statistical modeling to predict the impact of pricing.
As with B2C, we start with the same baseline for (automating) price optimisation, but there are some key notions to consider in this setting, and capabilities in the Data Cloud that lend themselves well to address these nuances:
Let us consider the scenario whereby a B2B retailer is presented a particular deal and is trying to identify the optimal pricing strategy. Through the use of the Data Cloud platform, this can be scored against peer groups and factoring multiple different signals into price recommendations e.g. strategy, deal size, customer type, and product type and mix.
Having established an optimal price point between B2B retailer and customer, how do we mature our demand forecasting to address the common B2B enquiry of “where is my stuff?”
Demand forecasting is one of the most difficult parts of B2B supply chain management, especially when you consider the number of factors that can affect it. It is also the most important, as all other pieces of supply chain management are based on predicted demand, including pricing, promotions and logistics optimisation. Many B2B retailers struggle with finding that balance when it comes to inventory, but transparency is an important strategy when building trust.
When we explored demand forecasting for B2C, there were three key influential factors:
For existing B2B relationships, historical transactions can often act as a reliable indicator of future demand/buying patterns - although not exclusively relied upon. We always have to factor an element of probability into our demand forecasting predictions.
In both cases, and similar to what was covered in Part 1and Part 2, data from a Customer Relationship Management (CRM) and/or Enterprise Resource Planning (ERP) system(s) can be ingested into the Data Cloud platform, before subsequently undergoing data pre-processing and used by Vertex Forecasting.
With the lack of speed of interactions (with suppliers) emerging as the number 1 pain point by many B2B customers, many B2B retailers also need to consider how they facilitate self-service and automation into existing processes. Some 86% of respondents in the same McKinsey B2B survey said they prefer using self-service tools for reordering, rather than talking to a sales representative. And whilst we haven’t covered the role ofpersonalised voice and chat bots in improving the B2B customer experience, or Google Cloud’s Document AI to automate data capture at scale, both are key adjacent technologies that are implicit parts of our combined AI/ML approach.
It is essential that the B2B retailer is not just empowered with the tooling and relevant insights to effectively apply pricing optimisation and demand forecasting, but to then automate, integrate and scale this across the relevant lines of the business. This allows them to use AI/ML to drive more business impact, and position the business for stronger ROI.
By using the Google Data Cloud, Kin + Carta can help B2B retailers close the data to value gap. We improve and speed up the decision-making process, while providing more granular insights. This accelerates value by breaking down data silos and leverage AI/ML to realise better outcomes, and enables better planning through demand forecasting, Inventory management, assortment planning, and dynamic pricing and promotions.
Want to know more about how Kin + Carta can help you on the road to a Sustainable Supply Chain?
Get in touch